Deep Fiber Clustering
- Yuqian Chen1,2
- Chaoyi Zhang 2
- Tengfei Xue1,2
- Yang Song 3
- Nikos Makris 1
- Yogesh Rathi 1
- Weidong Cai 2
- Fan Zhang 1
- Lauren J. O'Donnell 1
- 1Harvard Medical School
- 2University of Sydney
- 3University of New South Wales
Ourworks
Deep Fiber Clustering: Anatomically Informed Fiber Clustering with Self-supervised Deep Learning for Fast and Effective Tractography Parcellation (neuroimage 2023)
Deep Fiber Clustering: Anatomically Informed Unsupervised Deep Learning for Fast and Effective White Matter Parcellation (MICCAI 2021)
Overview
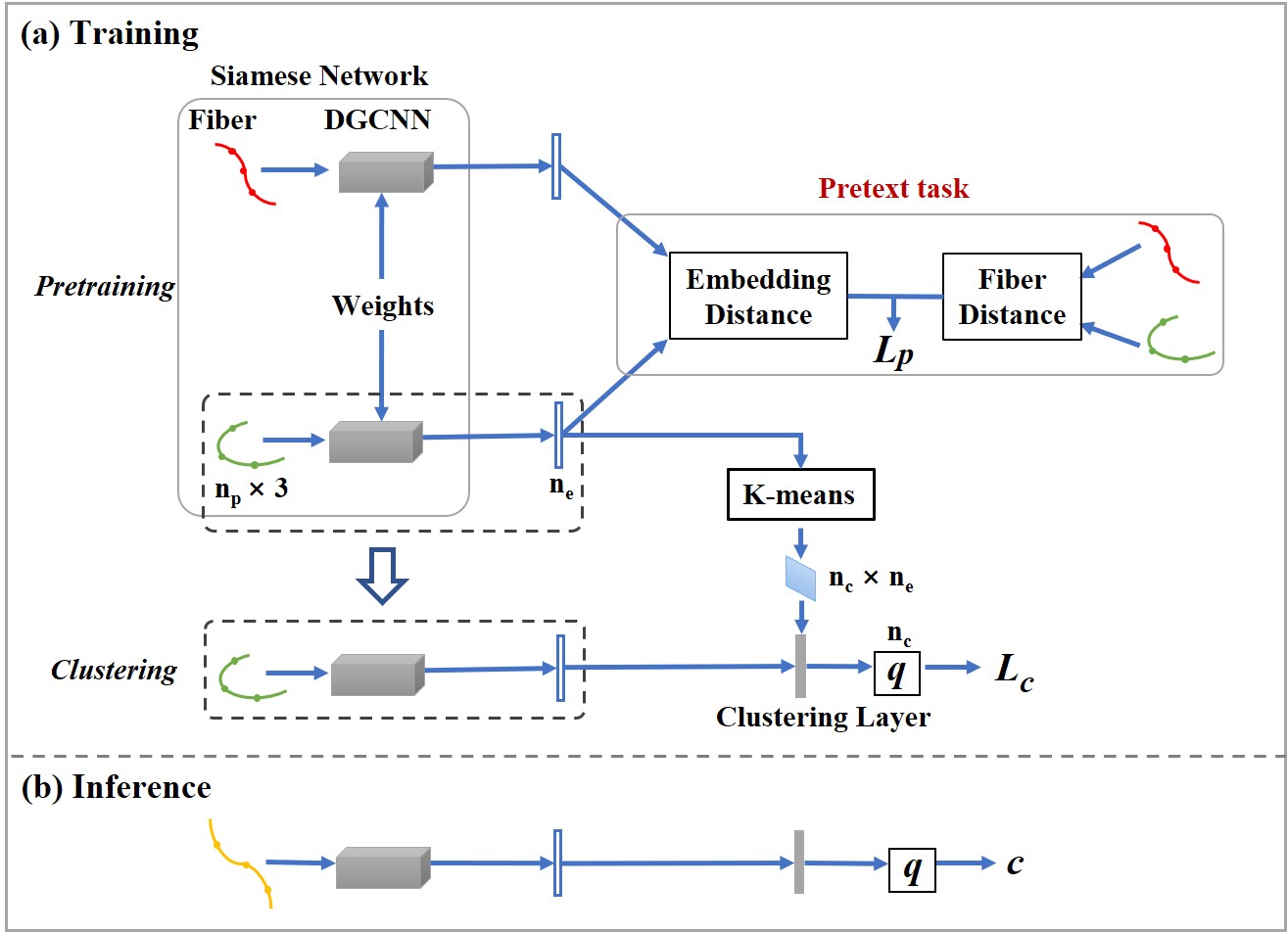
White matter fiber clustering (WMFC) is an important strategy for white matter parcellation, which enables quantitative analysis of white matter connections in health and disease. WMFC is usually performed in an unsupervised manner without the need of labeled ground truth data. While widely used WMFC approaches have shown good performance using classical machine learning techniques, recent advances in deep learning reveal a promising direction towards fast and effective WMFC. In this work, we propose a novel deep learning framework for WMFC, Deep Fiber Clustering (DFC), which solves the unsupervised clustering problem as a self-supervised learning task with a domain-specific pretext task to predict pairwise fiber distances. This enables the fiber representation learning to handle a known challenge in WMFC, i.e., the sensitivity of clustering results to the point ordering along fibers. We design a novel network architecture that represents input fibers as point clouds and allows the incorporation of additional sources of input information from gray matter parcellation. Thus DFC makes use of combined information about white matter fiber geometry and gray matter anatomy to improve anatomical coherence of fiber clusters. In addition, DFC conducts outlier removal in a natural way by rejecting fibers with low cluster assignment probability. We evaluate DFC on three independently acquired cohorts, including data from 220 individuals across genders, ages (young and elderly adults), and different health conditions (healthy control and multiple neuropsychiatric disorders). We compare DFC to several state-of-the-art WMFC algorithms. Experimental results demonstrate superior performance of DFC in terms of cluster compactness, generalization ability, anatomical coherence, and computational efficiency.
Video
(contains audio w/ subtitles)
Datasets
- Currently, training dataset is available upon request (email: fzhang@bwh.harvard.edu).
- Trained model and one test sample can be downloaded here.
BibTeX
If you find our project useful in your research, please cite:@article{chen2023deep, title={Deep Fiber Clustering: Anatomically Informed Fiber Clustering with Self-supervised Deep Learning for Fast and Effective Tractography Parcellation}, author={Chen, Yuqian and Zhang, Chaoyi and Xue, Tengfei and Song, Yang and Makris, Nikos and Rathi, Yogesh and Cai, Weidong and Zhang, Fan and O'Donnell, Lauren J}, journal={NeuroImage}, pages={120086}, year={2023}, publisher={Elsevier} } @inproceedings{chen2021deep, title={Deep Fiber Clustering: Anatomically Informed Unsupervised Deep Learning for Fast and Effective White Matter Parcellation}, author={Chen, Yuqian and Zhang, Chaoyi and Song, Yang and Makris, Nikos and Rathi, Yogesh and Cai, Weidong and Zhang, Fan and O’Donnell, Lauren J}, booktitle={International Conference on Medical Image Computing and Computer-Assisted Intervention}, pages={497--507}, year={2021}, organization={Springer} }